Department Systems Analysis, Integrated Assessment and Modelling
ML Tox
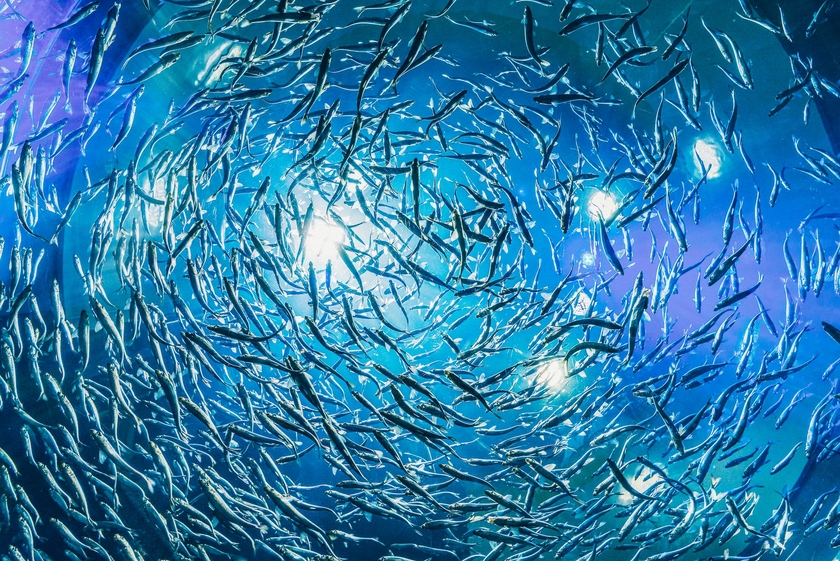
Photo by Harrison Haines from Pexels
We use machine learning methods to predict the effects of chemicals on aquatic species. Our main goal is to use a combination of data from in-vivo (whole organisms) and in-vitro (cell culture) experiments to infer the effects of chemicals on organisms for which no testing data is available (both for the chemical and for the organism). In the literature, this kind of problem is also known as across-chemical (and across-species) extrapolation. Usually, extrapolation across chemicals is performed using measures of chemical similarity under the assumption that similar chemicals will be similarly toxic to the same species. Extrapolation across species can be performed based on measured chemical effects on some species and the similarity between species, either by phylogenetic distance or sequence/structure similarity of known molecular targets of the chemicals, if at all available, or as well through similarity in physiological traits. Given the enormous number of chemicals and of potentially affected species, extrapolation chemical by chemical or species by species is a daunting task.
Our approach is different. In an interdisciplinary effort by ecotoxicologists and ML experts, we will combine thus far unconnected data to obtain predictions of toxicity across chemicals and species. ML allows the process to be agnostic to previously-determined notions of similarity between species or chemicals. We will use a variety of data sources and types, all available in different publicly available tools and databases, combining chemical structure, data on chemical testing on different organisms, and chemical testing in in-vitro assays.