Department Systems Analysis, Integrated Assessment and Modelling
Plankifier
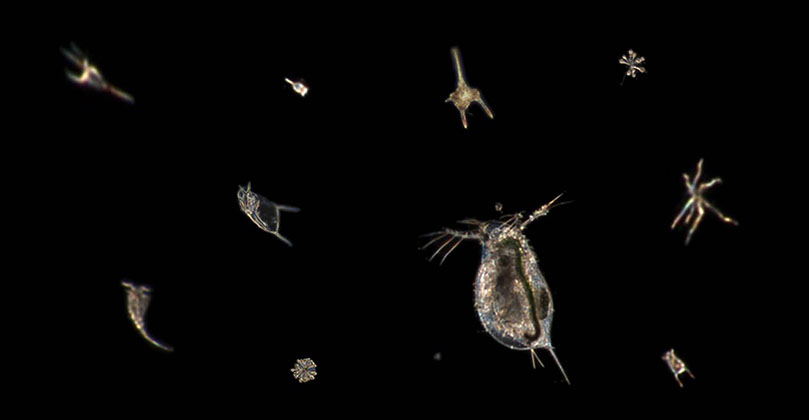
We test a big data workflow for understanding and predicting plankton dynamics using monitoring data. This is a timely case study, given the current streaming of plankton images
from automated monitoring, which need to be classified and analysed. Automated
classification of plankton pictures is challenging , due to the scarcity of labeled data for
training classifiers and the uneven frequency of detection among species. We propose a new
dataset construction and classification strategy based on semi-supervised, active, and
ensemble learning.
The outcoming classifiers are be used to create a large database of taxa abundances, their
features, and environmental conditions as a function of time. With this dataset, we test a
novel data- and modeling-oriented approach to cope with measurement choices, in such a
way that the modeling potential of the dataset is maximized. The whole workflow of the
proposal, from dataset construction and classification to data-oriented sampling, is explicitly
devised in order to be applicable to different kinds of environmental studies.