Department Systems Analysis, Integrated Assessment and Modelling
Process-based networks in hydrology
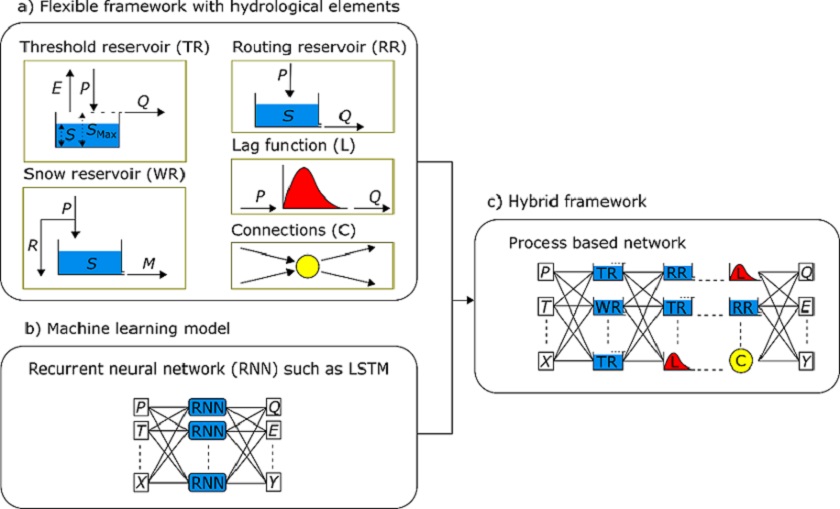
Catchment hydrology relies on various models, each with its own strengths and weaknesses regarding how easy they are to use, the data they need, and how well they predict outcomes. "Hybrid models" aim to combine the best features of different approaches to improve performance. A new area of research focuses on blending process-based (PB) models with machine learning (ML) models to enhance predictions and lead to new discoveries in hydrology.
This project builds on recent advancements in flexible conceptual models and combines them with the strengths of ML, especially deep learning, in streamflow prediction. The proposal introduces "process-based networks," where network nodes represent conceptual model elements, driven by mass conservation equations. These elements can represent different parts of the catchment, like unsaturated or saturated zones, and are more interpretable than the activation functions used in typical ML models.
The combination of flexible models and ML methods allows for efficient model calibration and parallel computing. We plan to run experiments on various catchments using attributes like topography and soil type, comparing our method to other models. The goal is to improve understanding of model complexity, catchment processes, and how to lean from the behavior of different catchments.
Funding
SNF